Machine Learning for enhancing road safety
With Road Accidents happening every minute, Our Country is in distress with severe losses of life and demolition of the property. Road Accidents do not occur by chance: It has patterns and can be examined, analysed, predicted and avoided. Being one of the most life-threatening incidents in the world, mitigating this severity and minimizing the occurrence of accidents is to be put into action.
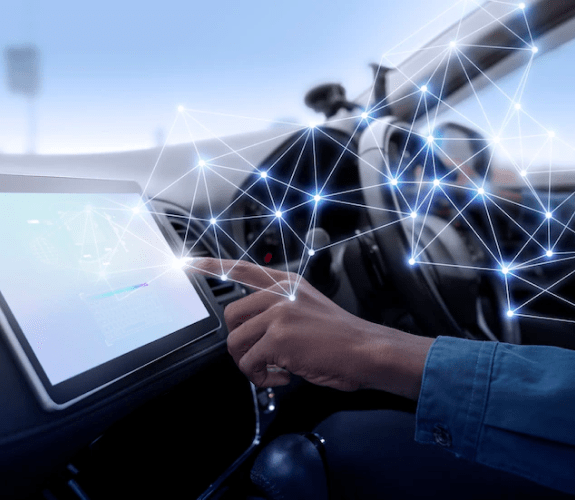
Case Studies:
Accidents could happen not only based on the expected parameters, but also under unanticipated circumstances. Predicting an occurrence leads to taking precautionary measures and hence reducing the risk of a casualty. The ML Model proves to be a reliable technique which can be used to analyse the data and to identify the major reasons which cause road accidents. This could be a major step in enhancing road safety in India. Data that is collected ranges from accident details, and road features to the traffic details of the past few years, we build a predictive Model, which gives a pattern on accident black spots, peak hour of the day and dependency on weather conditions. This model can be helpful in building a system for the prevention of traffic accidents and violations of law, the design of procedures for selecting a professional driver, alerting road users about accident black spots, and rehabilitation of drivers who have been deprived of their driving license.
We present an efficient framework for accident detection using computer vision and deep learning techniques to detect different types of trajectory conflicts including vehicle-to-vehicle, vehicle-to-pedestrian, and vehicle-to-bicycle. It aims to detect accidents in seconds by using advanced deep-learning algorithms that use convolutional neural networks (CNN or ConvNet) to analyze the frames from the real-time video generated by the traffic surveillance cameras. This detection system can be used as part of an emergency road call service that recognizes traffic accidents automatically and allows immediate rescue after transmission to emergency recovery agencies.